AIs exploit Fourier Duality of Information
In The Cosmic Computer I have suggested that the Universe consists of tw0 domains of information, each Fourier dual to the other, and separated in the dimension of pure scale at the level of atoms. The fact that the math of Fourier transforms that is a mainstay of information processing is identical to the 'weird' math of quantum mechanics is is a big clue as to why Nature builds such incredibly powerful IT into every atom, a single atom processing information faster than all the world's computers put together. That cosmic purpose is: communicating, processing and understanding data to create an optimum Universe.
Here are some recent discoveries of researchers about the role of Fourier transformed data in the latest advances in AI.

"For all their brilliance, artificial neural networks remain as inscrutable as ever. As these networks get bigger, their abilities explode, but deciphering their inner workings has always been near impossible. Researchers are constantly looking for any insights they can find into these models.
A few years ago, they discovered a new one.
In January 2022, researchers at OpenAI, the company behind ChatGPT, reported that these systems, when accidentally allowed to munch on data for much longer than usual, developed unique ways of solving problems. Typically, when engineers build machine learning models out of neural networks — composed of units of computation called artificial neurons — they tend to stop the training at a certain point, called the overfitting regime. This is when the network basically begins memorizing its training data and often won’t generalize to new, unseen information. But when the OpenAI team accidentally trained a small network way beyond this point, it seemed to develop an understanding of the problem that went beyond simply memorizing — it could suddenly ace any test data.
The researchers named the phenomenon “grokking,” a term coined by science-fiction author Robert A. Heinlein to mean understanding something “so thoroughly that the observer becomes a part of the process being observed.” The overtrained neural network, designed to perform certain mathematical operations, had learned the general structure of the numbers and internalized the result. It had grokked and become the solution." Anil Ananthaswamy, Quanta Magazine, 12-4-2024
So, rather than blindly memorizing data, an overtrained AI is able to reach a deeper, generalized understanding of the data, giving it much better accuracy and the ability to correctly process data that it has never seen in training. Although the inner workings of a neural network AI has been a complete mystery up until now, mathematicians have finally found some of the structures that an over-trained, 'grokking' AI creates for its data, and these depend on Fourier transforms of the original data.
This 2020 paper also shows how Fourier features improve the performance of deep, fully-connected neural networks in computer vision and graphics.
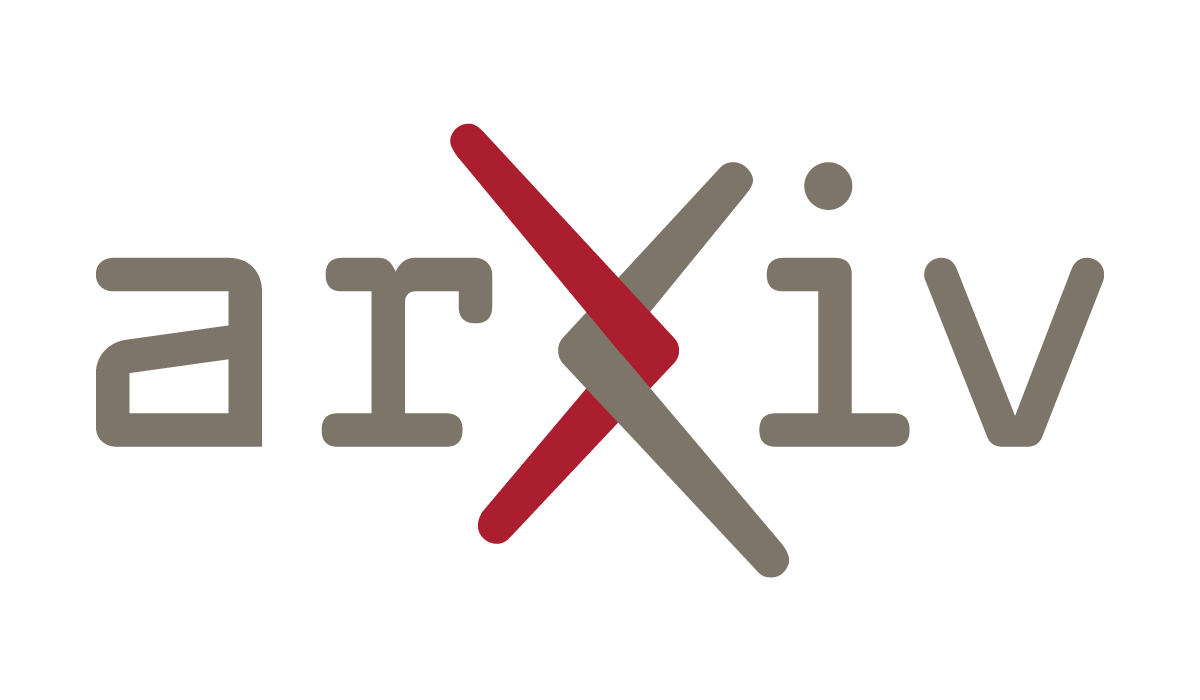